Asso. Prof. GUI Jie from Institute of Intelligent Machines (IIM), Chinese Academy of Sciences (CAS), has published a survey on sparse learning, titled Feature Selection Based on Structured Sparsity: A Comprehensive Study, in IEEE Transactions on Neural Networks and Learning Systems
(http://ieeexplore.ieee.org/xpl/RecentIssue.jsp?punumber=5962385).
The survey summarized the sparse learning and its application to feature selection, which is useful for the beginners to join into this area, and provided an in-depth understanding to this research field as well.
In many pattern recognition tasks, researchers are often confronted with very high-dimensional data. Feature selection(FS) algorithms are designed to identify the relevant feature subset from the original features, which can facilitate subsequent analysis, such as clustering and classification.
Structured sparsity-inducing feature selection (SSFS) methods have been widely studied in the last few years, and a number of algorithms have been proposed. However, there is no comprehensive study concerning the connections between different SSFS methods and how they have evolved.
Asso. Prof. GUI Jie attempts to provide a survey on various SSFS methods, including their motivations and mathematical representations. The survey explores the relationship among different formulations and proposes a taxonomy to elucidate their evolution. The existing SSFS methods are divided into two categories, i.e., vector-based feature selection (feature selection based on lasso) and matrix-based feature selection (feature selection based on r, p-norm). Furthermore, FS is combined with other machine learning algorithms for specific applications, such as multitask learning, multilabel learning, multiview learning, classification and clustering.
This survey not only compares the differences and commonalities of these methods based on regression and regularization strategies, but also provides useful guidelines to practitioners working in related fields.
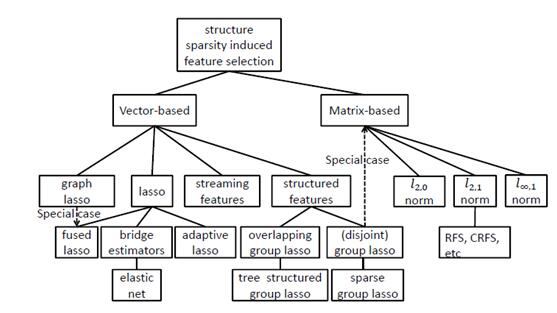
Figure 1. Taxonomy of structure sparsity-induced feature selection
The link of this paper: http://ieeexplore.ieee.org/document/7458185/.
The matlab code can be downloaded from: http://www.escience.cn/people/guijie/code.html.
Keywords: Sparse Learning, Feature Selection(FS), Structured Sparsity-Inducing Feature Selection (SSFS)
Contact:
Associate Prof. Jie Gui, Ph.D
Institute of Intelligent Machines,Chinese Academy of Sciences
( http://www.iim.cas.cn/)
Hefei, Anhui 230000, China
Tel:0551-65590621
E-mail:guijie@ustc.edu